Research
Giving clinicians the tools to tackle sepsis
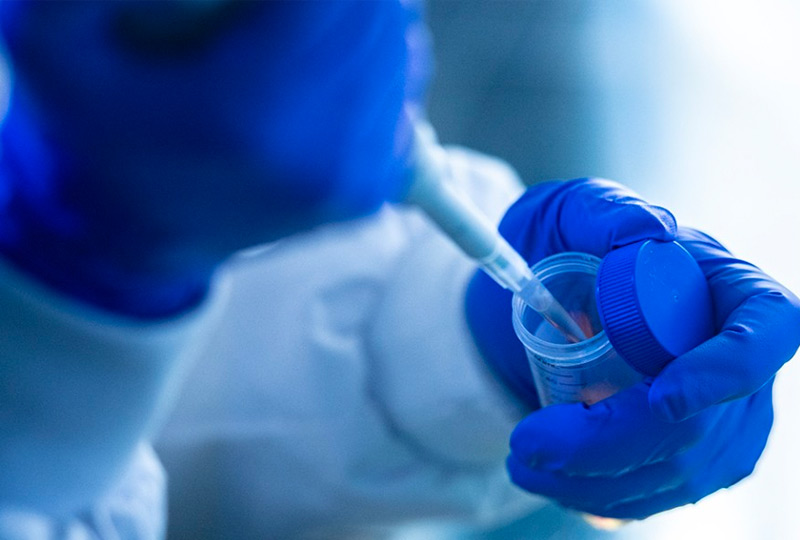
We are living through a period of profound technological change. Machine learning and AI could transform many aspects of our lives, which explains why governments and tech companies are so focused on these fields.
Some of the most exciting opportunities are emerging in biotech and healthcare. We are moving towards personalised medicine and data-driven decision making. New tools can support healthcare workers, especially in complex medical scenarios involving difficult choices.
At GenomeKey, we have honed in on one life-threatening condition: sepsis. This occurs when the body’s immune system reacts severely to an infection, potentially leading to septic shock, where blood pressure plummets, bringing a risk that multiple organs fail. In most cases, sepsis occurs as a complication of bacterial infections such as pneumonia, urinary tract infections, or infections of the bloodstream.
Tackling uncertainties
Speed is essential in treating sepsis and antibiotics are currently our primary form of defence when the cause is bacterial. In cases of septic shock, for every hour that antimicrobial treatment is delayed, survival rates drop by 7.6%. In an ideal world, doctors would instantly know the pathogen causing the sepsis and which antibiotic will be effective.
Right now, medics rely on blood cultures. In these diagnostic tests, a patient blood sample is taken to a lab where conditions are optimised for microbe growth. Then it’s a waiting game – often spanning several days – to figure out what bacteria might be present and how it responds to specific antibiotics. This is far too long to wait for patients with severe sepsis, so doctors administer broad spectrum IV antibiotics to fight the infection during this period, which can have side effects.
Even if blood cultures were much faster, they still contain uncertainties. Insufficient blood samples can lead to false negatives, therefore multiple tests over a period are often required. Factors such as sample handling, transportation, and processing can all affect the accuracy of the test. And just because bacteria succumb to an antibiotic in a petri dish, doesn’t necessarily mean they will do the same in the body.
Rather than offer incremental improvement, GenomeKey has devised a completely different approach to bacterial diagnostics. We are developing a benchtop device that leverages whole genome sequencing and can discover bacteria’s antibiotic resistance (AMR) profile within a couple of hours with the help of AI. Doctors faced with a bacterial sepsis case will be able to pinpoint the best course of antibiotics quickly and with confidence.
Understanding bacterial behaviour
Because we use whole genome sequencing, we can analyse bacteria’s antibiotic resistant qualities more comprehensively, which may not be evident in conventional AMR procedures. For instance, bacteria can produce inhibitors in the form of proteins, enzymes, or molecules that bind to the antibiotic and prevent it from reaching its target in the bacterial cell.
Another challenge is that bacteria can be protected behind a robust layer (a biofilm) that blocks antibiotics. Knowing a bacteria’s genome means you can spot these traits even if the bacteria has not yet expressed them.
Of course, vast amounts of data are only useful if presented in a meaningful way. Our machine learning algorithms handle the data analysis to present clinicians with clear insights, which can inform treatment decisions. This could mark another step-change in medicine, just like the introduction of X-ray imaging in the 20th century, giving clinicians an advanced tool to leverage for data-driven medical support.
Sepsis is our initial application area because the needs and impact are so clear. But as our technology can detect and identify absolutely any bacteria, the potential applications are vast. It could help to identify localised outbreaks of hospital-acquired superbugs including Methicillin-resistant Staphylococcus aureus (MRSA) and Clostridioides difficile (C. diff). Over the longer term this data could feed into national monitoring systems for emerging antibiotic resistance trends and patterns.